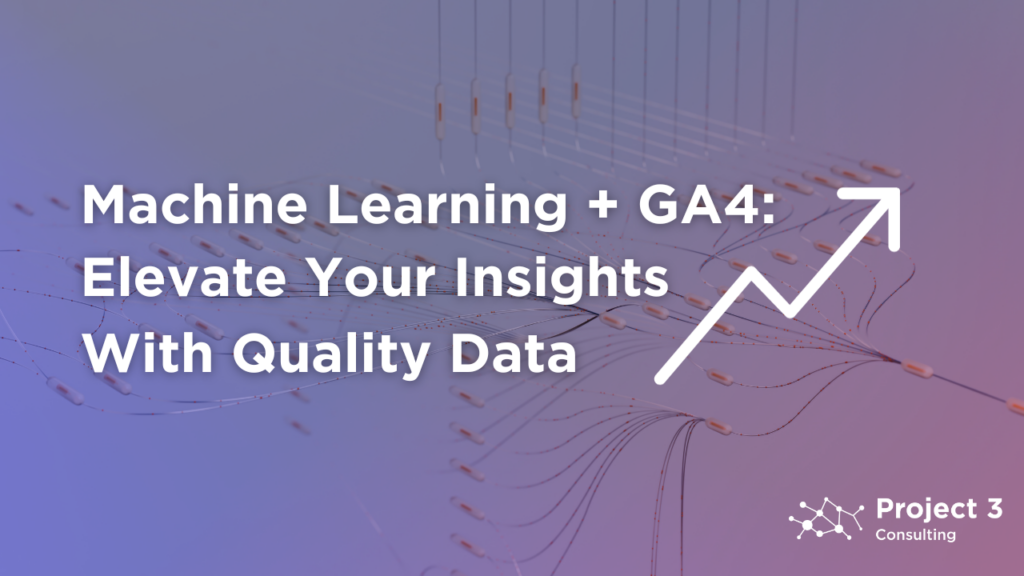
Google Analytics 4 (GA4) is a powerful digital analytics platform that leverages machine learning (ML) to provide businesses with valuable insights. While GA4’s ML capabilities are well-publicized (and can do some pretty cool things), it’s also important to understand how ML functions in the context of digital analytics, along with the role of data quality and structuring to optimize GA4’s performance.
(Keep reading to the end to learn some practical examples of how to improve data hygiene using Google Tag Manager.)
What is Machine Learning in Digital Analytics?
Machine learning refers to the utilization of advanced algorithms to analyze large datasets and discover patterns, trends, and anomalies that might be challenging to identify through traditional analytical methods. ML algorithms in digital analytics, such as those employed by GA4, are designed to automatically adapt and improve their performance over time without being explicitly programmed.
These algorithms learn from historical data, adjusting their models to deliver more accurate and actionable insights. In the context of GA4, machine learning is used to predict user behavior, analyze conversion paths, and offer recommendations based on a website’s data. This enables businesses to make informed decisions, refine their digital strategies, and ultimately enhance their online presence.
So, what do you need to do from a data collection perspective in a world where ML is integrated into your analytics tools? It all boils down to two words: Data Quality.
Garbage In, Garbage Out
GA4 relies on machine learning to offer meaningful insights, projections, and recommendations. However, what is often forgotten is the fact that the quality of your input data directly influences the quality of the insights it generates. Think of GA4 as a powerful machine running on data – cleaner, structured data acts as premium fuel, allowing GA4’s ML algorithms to perform at their best, resulting in more accurate insights and reliable projections.
Or to put it more succinctly: garbage in, garbage out.
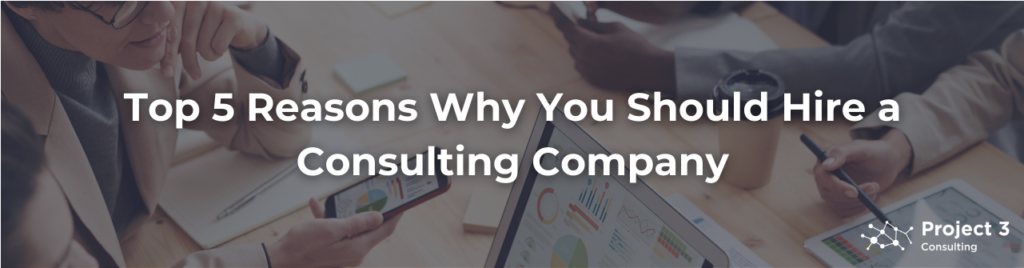
Maintaining Data Hygiene
Maintaining good data hygiene is crucial for making the most of GA4. Regular data cleaning, ensuring data consistency, and eliminating duplicates not only improve report accuracy but also enhance GA4’s ML models. Clean data is essential to prevent erroneous conclusions and ensure data-driven marketing teams can excel in their roles.
And like any hygiene practice, this is not something you can do once and forget about it – it is an ongoing task, and problems can get worse the longer you neglect them.
Structuring for Success
Organizing your data in a structured manner is another key factor in optimizing GA4’s performance. Well-structured data is easier for GA4’s ML algorithms to process, resulting in faster and more precise insights. When you structure your data logically, you facilitate the model’s ability to identify patterns and trends.
In other words, feed the machine well and it’ll feed you back with better insights.
This means you’ll want to ensure consistency in event names and parameters and/or make use of Google’s suggestions. Another way to ensure sound structuring is to implement a robust data layer to pass data payloads when the user takes actions on the site rather than sending information to analytics by page scraping.
The Result? Maximized Insights and Recommendations
Clean, structured data isn’t just about improving the accuracy of your reports for some nameless, faceless board member to review; it’s about elevating your decision-making capabilities and taking smarter actions to drive returns on investment. When GA4’s ML model operates on quality data, the insights and recommendations it provides become more actionable and reliable.
This, in turn, empowers you to make informed decisions that drive business growth. Win, win.
How To: Front-load Data Hygiene with GTM
Front-loading the data hygiene process can significantly enhance GA4’s reliability. One effective method is to use Google Tag Manager (GTM) to clean and structure your data before it reaches GA4. Here are some examples of how to do this:
Lookup Table Variable: Use GTM’s Lookup Table Variable to map messy or inconsistent data to standardized values.
- If your Country data contains variations like “US,” “USA,” and “United States,” you can use a Lookup Table to unify them as “United States”
- If you want to exclude your team’s debugging traffic from GA4, you can use a Lookup Table to filter out this traffic – this means that no test data enters the system (and trains the algorithms) while you’re testing your implementation
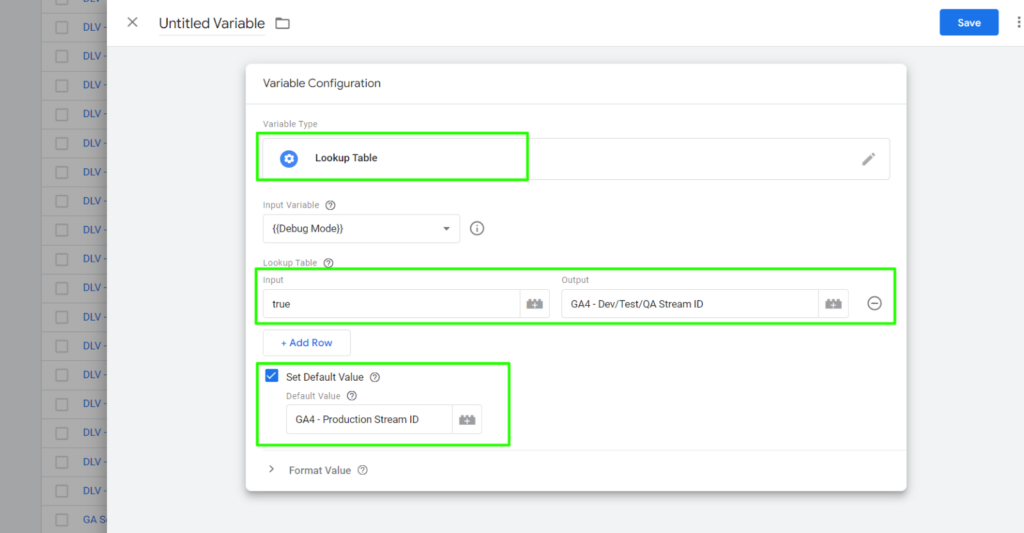
Regular Expression Table Variable (RegEx): Implement RegEx Table Variables in GTM to extract specific information from messy strings.
- You can use RegEx to extract product IDs from URLs, ensuring that GA4 receives clean, structured data.
- You can remove developer, UAT, and staging traffic from your production data stream by using the Page Hostname as an input variable and directing all non-production traffic to the non-production GA4 property.
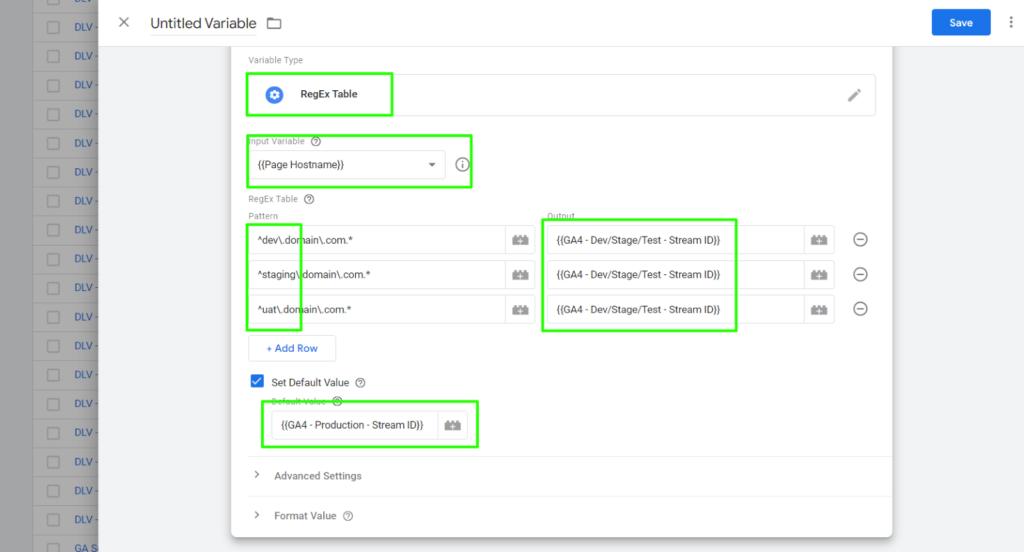
By frontloading the data hygiene process via GTM, you ensure that the data flowing into GA4 is already in optimal condition, reducing the need for extensive post-processing during analysis and removing bad data from ML processes.
It All Comes Down to Quality Data
In the world of digital analytics, Google Analytics 4 stands as a powerful tool. However, to truly harness its capabilities and unleash the full potential of its machine learning algorithms, we need to be talking about and practicing data cleansing, hygiene, and structuring. Investing in clean, structured data is critical to ensure that GA4 provides you with the reliable insights, projections, and recommendations you need to trust your data. Plus, by front-loading the data hygiene process with GTM, you can streamline your data pipeline and make the most of GA4 from the very beginning. So, remember, when it comes to GA4, it’s not just about what you put in; it’s about putting in the best to get the best out.
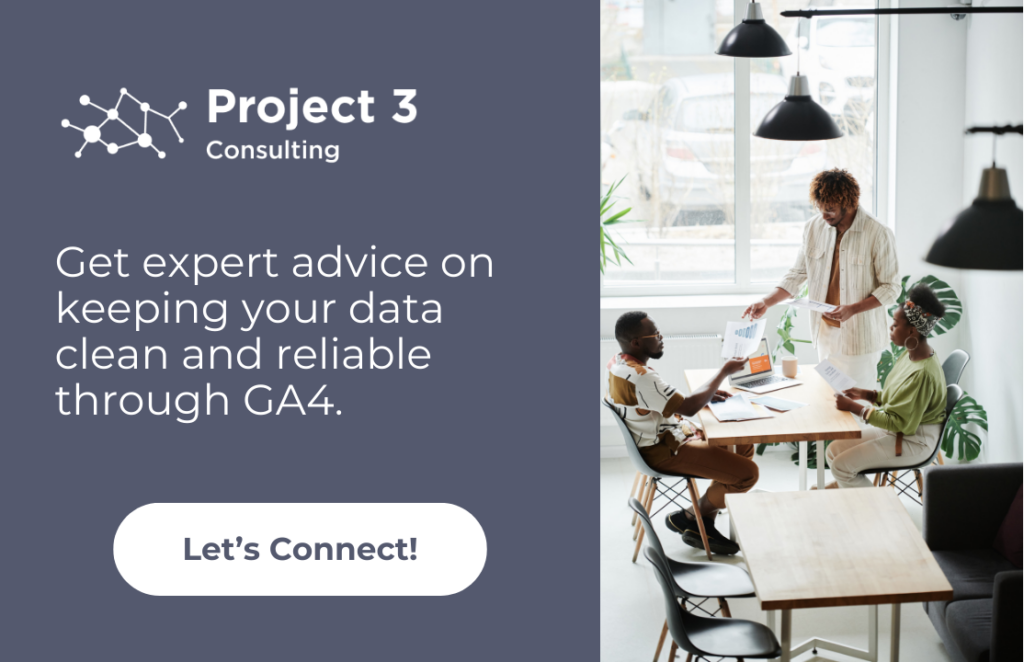